
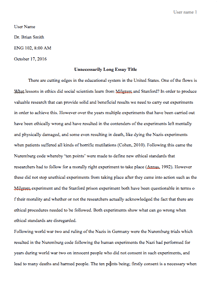
ABSTRACT
Marketing data in the banking sector is an example of Big Data considering that they are available in large volumes with a steadily growing base due to the changing nature of the financial sector. In the banks' marketing, the application of data mining in the classification of marketing data has the objective of allowing banks to enhance their sales, marketing, and support customer operation by improving CRM how they understand their customers. Since classification is the most popular data mining tactics, the paper explores common data classification tools including Neural Networks, Decision Trees (DT), SVM, Naive Bayes and Logistic Regression with the aim of discovering a preferred classification model among different banks.
1. INTRODUCTION
In the contemporary business world, CRM-data mining is gaining popularity due to its role in establishing and managing close relationships between a business organization and their customers. By definition, data mining is the application of artificial intelligence, statistical, mathematical or machine learning strategies for identification and extracting of valuable information from various databases. Classification is the conventional data mining technique applicable in the process of predicting customer behaviors hence enabling business organizations in enhancing their decision making CITATION Pra10 \l 1033 (Koturwar, Girase, & Mukhopadhyay, 2010). Banks are currently applying classification models as a data mining strategy for CRM-based bank marketing applications like risks analysis, profitability, customer identification, segmentation, attraction, development, and retention. The success of a classification model as a data mining strategy in banks is customer retention and the ability to pinpoint prospects by marketing additional services. Classifying data remains of the preferred data mining learning model in marketing for the prediction of customer behaviors, forecasting and decision making. Marketing prediction using classification entails categorizing customer records in various classes depending on given criteria (Radhakrishnan, Shineraj, & Muhammed, 2013, p. 43). The resultant information from data processing are applicable in CRM-data mining uses such as retention of valued clients.
A large number of banks marketing campaigns have lost their effectiveness to the general public due to poor classification strategies which lead to the banks succumbing to competition and economic pressures. One of the typical survival tactics among the banks marketing managers is the investment on directed marketing campaign strategies with the classification technique being the rigorous and strict selection of customer and clients contacts. There is a likelihood of enhancing the effectiveness of the direct campaigns through the application of data mining and business intelligence techniques. A bank can market or promote its services and products in two ways. The first one is through mass campaigning which does not discriminate on the target audiences and the second strategy is the application of direct marketing in which the bank target a particular population using a set of customer contacts. There is an increasing wave of shift by financial institutions such as banks from mass campaigns to direct marketing due to the effectiveness of the later. Data classification is essential in targeting the customers for the direct marketing for specific banking services and products. As Elsalamony and Elsayad (2013) suggest, one of the excellent strategies for improving the efficiency of the business campaigns is the use of data mining which in particular, utilize data mining classification approaches in building predictive models that label data items and classify them into distinct classes. For the ranking of marketing contacts for direct marketing in the banking sector, the application of data mining classification technique will depend on purpose and capability. The data mining classification techniques that can enhance data classification include Support Vector Machine (SVM), Decision Trees (DT), Naive Bayes (BT), and Neural Networks (NN).
2. PROBLEM STATEMENT
Despite the presence of different data classification models, there is an acute difference in metrics specificity, sensitivity analysis, and accuracy rate. Therefore, the research will recommend an efficient classification as data mining model that can bring effective results in CRM-based banking marketing applications such as predicting customer behaviors, customer identification, segmentation, retention, and development.
2.1 Research Question
Which is an appropriate CRM-data mining strategy that a bank can apply in enhancing the direct marketing applications?
2.2 Hypothesis
The research has the basis of an affirmative proposition that a suitable data classification technique, should assist banks in identification, segmentation, development and retention of valuable customers and predict future behaviors.
3. RESEARCH OBJECTIVES
3.1 Overall Objective
The study has the sole purpose of offering an integrative review of an appropriate CRM-data mining model basing on data classification that can produce useful results in applying banks telemarketing data for various customer-related applications.
3.2 Specific Aims
The secondary objectives of the research that facilitate the achievement of the primary purpose include
To study and evaluate different data mining classifier models
To apply banks marketing data sets in assessing performance of different classifiers basing on classification metrics such as specificity, sensitivity analysis, and accuracy rate
4. BACKGROUND AND SIGNIFICANCE
Almost every bank have vast records of customer data. Due to the widespread application of stored data in direct marketing, such enormous customer data can be useful for the creation and maintenance of connection and relationship with their clients. Classification of such data is helpful for targeting the customers and cataloging them basing on specific services and products which the financial institution offer. The classification technique for bank marketing should select and classify customers basing in through variables such as email, mail, telephone cellular, personal contacts another available means that the banks can reach the target customers to advertise their services and products which are the essence of direct marketing. The concept of direct marketing has, in the recent past, become common among insurance companies and other financial institution for establishing a platform for interacting with clients and customers. Data mining is the most useful intelligent system and strategy that aid banks in the process of classifying that data and extracting predictive, explanatory and helpful insight from the raw banking data. Particularly, data classification is one of the most common data mining tasks that any bank considers in its attempt to classify data and catalog the customers to establish a data-driven prototypical system that has the capability of characterizing the client variables and learning their banking behavior.
The significance of the research is that it will lead to the proposition of an active CRM-data mining tactic in the form of classification framework for comprehending the banks marketing goals and solution in data mining perspective. Data mining has the reputation of maintaining a close relationship with CRM since it avail data necessary for identifying customer pattern and predict future behaviors. Data classification deals with preprocessing and preparation of data for selection and classification of attributes, cleaning, and transformation for the advance development of CRM-data mining simulations. One of the importance of the exploration of the data classification techniques is that it can assist bank managers in rethinking marketing the study can enable them to focus on the appropriate data classification and handling strategies that can maximize the lifetime value of the esteem customers. Furthermore, integration of the insights from the study into the mainstream marketing activities of a bank can assist the financial institution to evaluate customer metrics and available information hence building tighter and stronger customer relations according to the banks business demands.
5. Literature Review
Previous studies such as that of Han and Kamber (2012) define data mining as the process of transforming raw data to produce results that are actionable. Data classification is a form of data mining strategies for organizing amorphous data into classes of organized data. Chen (2010) suggests that the success of the data mining process do not involve the application of right tools and algorithms but entail how an organization can derive business sense from the stored data. Well classified data are useful, but the redundancy of data in storage points before application represent one of the pitfalls that undermine the success of the data mining procedure. Data classification in financial sector faces numerous problems such as storage in inappropriate data formats, and application of data fields that are confusing. Banks' marketing data are examples of big data due to the constant generation of data from the field that keeps on mounting every second (Fong & Yang, 2011). One of the leading aspects of the establishment of an effective CRM framework in data mining is the comprehension, analysis, and prediction of customer behavior which have the sole purpose of boosting the acquisition and retention of potential customers for maximization of their value (Bahari & Elayidom, 2015, p. 725). Classification as the standard CRM-data mining strategy has the reputation of assisting organizations in the identification and prediction of future customer behaviors CITATION Yen14 \l 1033 (Yenkar & Bartere, 2014). The effectiveness of banks marketing data is reliant on the efficiency, accuracy, specificity and sensitivity of the data mining strategy applied. There exist a wide variety of classification techniques in data mining which include SVM, logistic regression, naive Bayes, Decisi...
Request Removal
If you are the original author of this essay and no longer wish to have it published on the customtermpaperwriting.org website, please click below to request its removal: